Entropy and Mutual Information
I’m interested in looking at some spatial mappings between pairs of cortical regions, and believe that these mappings are mediated, to some degree, by the temporal coupling between cortical areas. I don’t necessarily know the functional form of these mappings, but neurobiologically predict that these mappings are not random and have some inherent structure. I want to examine the relationship between spatial location and strength of temporal coupling. I’m going to use mutual information to measure this association.
It’s been a while since I’ve worked with information-based statistics, so I thought I’d review some proofs here.
Entropy
Given a random variable $X$, we define the entropy of $X$ as
$$\begin{align} H(X) = - \sum_{x} p(x) \cdot log(p(x)) \end{align}$$
Entropy measures the degree of uncertainty in a probability distribution. It is independent of the values $X$ takes, and is entirely dependent on the density of $X$. We can think of entropy as measuring how “peaked” a distribution is. Assume we are given a binary random variable $Y$
$$\begin{align}
Y \sim Bernoulli(p) \\
\end{align}$$
such that
$$\begin{align}
Y =
\begin{cases}
1 & \text{with probability $p$} \\
0 & \text{with probability $1-p$}
\end{cases}
\end{align}$$
If we compute $H(Y)$ as a function of $p$ and plot this result, we see the canonical curve:
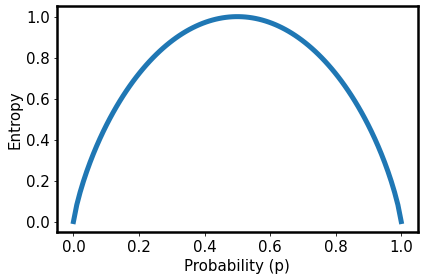
Immediately evident is that the entropy curve peaks when $p=0.5$. We are entirely uncertain what value $y$ will take if we have an equal chance of sampling either 0 or 1. However, when $p = 0$ or $p=1$, we know exactly which value $y$ will take – we aren’t uncertain at all.
Entropy is naturally related to the conditional entropy. Given two variables $X$ and $Y$, conditional entropy is defined as
$$\begin{align}
H(Y|X) &= -\sum_{x}\sum_{y} = p(x,y) \cdot log(\frac{p(x,y)}{p(x)}) \\
&= -\sum_{x}\sum_{y} p(y|x) \cdot p(x) \cdot log(p(y|x)) \\
&= -\sum_{x} p(x) \sum_{y} p(y|X=x) \cdot log(p(y|X=x))
\end{align}$$
where $H(Y|X=x) = -\sum_{y} p(y|X=x) \cdot log(p(y|X=x))$, the conditional of entropy of $Y$ given that $X=x$. Here, we’ve used the fact that $p(x,y) = p(y|x) \cdot p(x) = p(x|y) \cdot p(y)$.To compute $H(Y|X)$, we take the weighted average of these conditional entropies, where weights are defined by the marginal probabilities of $X$.
Mutual Information
Related to entropy is the idea of mutual information. Mutual information is a measure of the mutual dependence between two variables. We can ask the following question: does knowing something about variable $X$ tell us anything about variable $Y$?
The mutual information between $X$ and $Y$ is defined as:
$$\begin{align}
I(X,Y) &= \sum_{x}\sum_{y} p(x,y) \cdot log\Big(\frac{p(x,y)}{p(x) \cdot p(y)}\Big) \\
&= \sum_{x}\sum_{y}p(x,y) \cdot \log(p(x,y)) - \sum_{x}\sum_{y}p(x,y) \cdot log(p(x)) - \sum_{x}\sum_{y}p(x,y) \cdot log(p(y)) \\
&= -H(X,Y) - \sum_{x}p(x) \cdot log(p(x)) - \sum_{y}p(y) \cdot log(p(y)) \\
&= H(X) + H(Y) - H(X,Y)
\end{align}$$
$I(X,Y)$ is symmetric in $X$ and $Y$:
$$\begin{align}
I(X,Y) &= \sum_{x}\sum_{y} p(x,y) \cdot log\Big(\frac{p(x,y)}{p(x) \cdot p(y)}\Big) \\
&= \sum_{x}\sum_{y} p(x,y) \cdot log\Big(\frac{p(x|y)}{p(y)}\Big) \\
&= \sum_{x}\sum_{y} p(x,y) \cdot log(p(x|y)) - \sum_{x}\sum_{y}p(x,y) \cdot log(p(x)) \\
&= -H(X|Y) - \sum_{x}\sum_{y} p(x|y) \cdot p(y) \cdot log(p(x)) \\
&= -H(X|Y) - \sum_{x}log(p(x) \sum_{y}p(x|y) \cdot p(y) \\
&= -H(X|Y) - \sum_{x} p(x) \cdot log(p(x)) \\
&= H(X) - H(X|Y) \\
&= H(Y) - H(Y|X)
\end{align}$$
We interpret the above to mean the following: if we are given any information about X (Y), can we reduce the uncertainty around what Y (X) should be? We understand how much variability there is in each variable independently – this is measured by the marginal entropy $H(Y)$. If knowing $X$ reduces this uncertainty, then the conditional entropy $H(Y|X)$ should be small. If knowing $X$ does not reduce this uncertainty, then $H(Y|X)$ can be at most as large as $H(Y)$, and we have learned nothing about our dependent variable $Y$.
Put another way, if $I(X,Y) = H(Y) - H(Y|X)$ is large, then the mutual information between $X$ and $Y$ is large, indicating that $X$ is informative of $Y$. However, if $I(X,Y)$ is small, then the mutual information is small, and $X$ is not informative of $Y$.
Application
For my problem, I’m given two variables, $Z$ and $C$. I’m interested in examining how knowledge of $C$ might reduce our uncertainty about $Z$. $C$ itself is defined by a pair of variables, $A$, and $B$, such that we have $C_{1} = (a_{1}, b_{1})$. $Z$ is distributed over the tensor-product space of $A$ and $B$, that is:
$$\begin{align} Z = f(A \otimes B) \end{align}$$
where $A \otimes B$ is defined as
$$\begin{align}
A \otimes B =
\begin{bmatrix}
(a_{1},b_{1}) & \dots & (a_{1},b_{k}) \\
\vdots & \ddots & \vdots \\
(a_{p}, b_{1}) & \dots & (a_{p}, b_{k})
\end{bmatrix}
\end{align}$$
such that $z_{i,j} = f(a_{i}, b_{j}$)
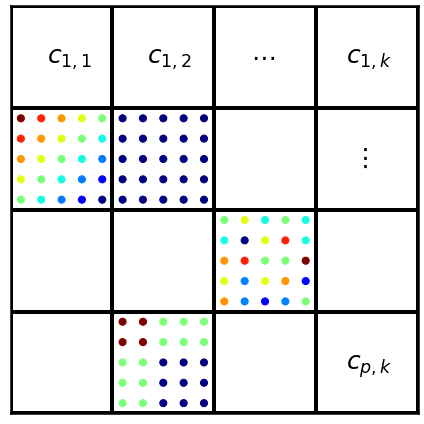
We define the mutual information between $Z$ and $C$ as
$$\begin{align}
I(Z,C) &= \sum_{z}\sum_{c} p(z,c) \cdot log\Big(\frac{p(z,c)}{p(z)p(c)} \Big) \\
&= H(Z) - H(Z|C) \\
&= H(Z) - H(X|(A,B)) \\
&= H(Z) - \sum_{a,b} p(a,b) \sum_{z} p(z|(A,B)=(a,b)) \cdot log(p(z|(A,B)=(a,b)))
\end{align}$$
where the pair $(a,b)$ represents a bin or subsection of the tensor-product space. The code for this approach can be found below:
import numpy as np
def entropy(sample):
"""
Compute the entropy of a given data sample.
Bins are estimated using `Freedman Diaconis` Estimator.
Args:
sample: float, NDarray
data from which to compute entropy of
Returns:
H: entropy
"""
if np.ndim(sample) > 1:
sample = np.reshape(sample, np.product(sample.shape))
edges = np.histogram_bin_edges(sample, bins='fd')
[counts,_] = np.histogram(sample, bins=edges)
# compute marginal distribution of sample
m_sample = counts/counts.sum()
return (-1)*np.nansum(m_sample*np.ma.log2(m_sample))
def mutual_information_grid(X,Y,Z):
"""
Compute the mutual information of a dependent variable over a grid
defined by two indepedent variables.
Args:
X,Y: float, NDarray
coordinates over which dependent variable is distributed
Z: float, array
dependent variable
Returns:
estimates: dict
keys:
I: float
mutual information I(Z; (X,Y))
W: float, NDarray
matrix of weighted conditional entropies
marginal: float
marginal entropy
conditional: float
conditional entropy
"""
x_edges = np.histogram_bin_edges(X, bins='fd')
[xc, _] = np.histogram(X, bins=x_edges)
xc = xc/xc.sum()
nx = x_edges.shape[0]-1
y_edges = np.histogram_bin_edges(Y, bins='fd')
[yc, _] = np.histogram(Y, bins=y_edges)
yc = yc/yc.sum()
ny = y_edges.shape[0]-1
# matrix of conditional entropies for each bin
H = np.zeros((nx, ny))
# compute pairwise marginal probability of X/Y bins
mxy = xc[:,None]*yc[None,:]
for x_bin in range(nx):
for y_bin in range(ny):
x_idx = np.where((X>=x_edges[x_bin]) & (X<x_edges[x_bin+1]))[0]
y_idx = np.where((Y>=y_edges[y_bin]) & (Y<y_edges[y_bin+1]))[0]
bin_samples = Z[x_idx,:][:,y_idx]
bin_samples = np.reshape(bin_samples,
np.product(bin_samples.shape))
H[x_bin, y_bin] = entropy(bin_samples)
W = H*mxy
conditional = np.nansum(W)
marginal = entropy(Z)
I = marginal - conditional
estimates = {'mi': I,
'weighted-conditional': W,
'marginal': marginal,
'conditional': conditional}
return estimates